
Prognostics and health management oriented data analytics suite for transformer health monitoring
The correct operation of a power transformer depends on the correct operation of multiple related components over a variety of conditions.
byJose I. Aizpurua, Brian G. Stewart, Stephen D. J. McArthur
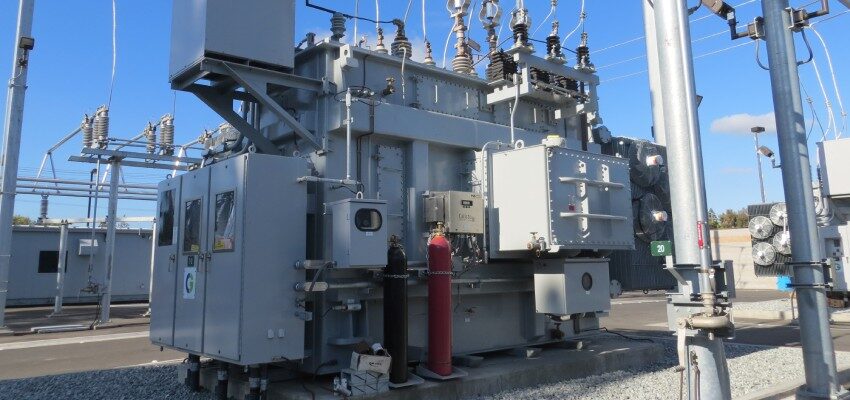
The correct operation of a power transformer depends on the correct operation of multiple related components over a variety of conditions. When monitoring the transformer’s health, the possible failure modes need to be examined. Traditional methods for transformer health assessment have been focused on the analysis of, e.g. gases dissolved in oil, temperature, or electrical parameters [1]. With the advance of machine learning (ML) techniques, traditional transformer health monitoring techniques can be enhanced with the development of prognostics and health management (PHM) applications, including anomaly detection, diagnostics, and prognostics analysis modules [2]. For a consistent implementation of ML methods for transformer health monitoring, a multistage PHM-oriented methodology is needed. Fig. 1 shows the block diagram of the PHM-oriented design methodology.
The PHM-oriented analytics process starts from the data audit step by listing available datasets and identifying new variables that can be monitored to improve the health assessment process. Next, correlation and anomaly detection are implemented to identify abnormal dana patterns. If an anomalous data trend is detected, then the diagnostics follows the process for the identification of failures. After diagnosing the current health, it is possible to implement prognostics methods to estimate the remaining useful life (RUL) through the application of future operation profiles.